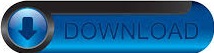
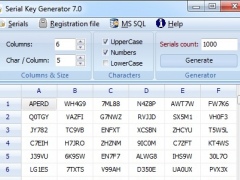
- INPAINT 6.0 KEY HOW TO
- INPAINT 6.0 KEY SERIAL KEY
- INPAINT 6.0 KEY GENERATOR
- INPAINT 6.0 KEY PATCH
- INPAINT 6.0 KEY SOFTWARE
Typically, the generated distribution of data is embedded in a low-dimensional manifold. Generative models are a popular tool in machine learning to generate data whose properties closely resemble those of a given database. Recently, Bayesian inverse problems were solved using generative models as highly informative priors. This critical ingredient allows the formulation of expert knowledge or physical constraints in a probabilistic fashion and plays an important role for the success of the inference. The Bayesian approach to solving inverse problems relies on the choice of a prior. We show ConvNPs can outperform classical methods and state-of-the-art deep learning inpainting models on a scanline inpainting problem for LANDSAT 7 satellite images, assessed on a variety of in and out-of-distribution images. In this work, we cast satellite image inpainting as a natural meta-learning problem, and propose using convolutional neural processes (ConvNPs) where we frame each satellite image as its own task or 2D regression problem. However, many of these methods do not explicitly take into account the inherent spatiotemporal structure of satellite images. Inpainting involves predicting what is missing based on the known pixels and is an old problem in image processing, classically based on PDEs or interpolation methods, but recent deep learning approaches have shown promise. For example, the scanline corrector for the LANDSAT 7 satellite broke down in 2003, resulting in a loss of around 20\% of its data. However, many satellite images have missing values due to measurement defects, which render them unusable without data imputation.


The widespread availability of satellite images has allowed researchers to model complex systems such as disease dynamics. The experiments conducted on Celeba dataset proved that our proposed algorithm quantitatively and qualitatively outperformed the baselines. Thereby, a lot of training time was saved. Finally, by finding the minimum data set with similar network expression ability, we quickly obtained the optimal value of multi-head.
INPAINT 6.0 KEY GENERATOR
Thirdly, a multi-head attention mechanism was introduced into the generator and local discriminator to build a multilevel and multi-dimensional dependent network model for image subspaces, which improved the global consistency of the inpainted image. Secondly, we designed a patch-GAN as the local discriminant to capture high frequency, and a function L2-loss was utilized to keep the high resolution and style of the original image. Firstly, an edge detector was introduced into the generator of multi-scale generative adversarial networks (GAN) to guide the inpainting of the edge contour in the image inpainting, which improved the inpainting effect of image posture and expression.
INPAINT 6.0 KEY PATCH
In this paper, the multi-scale patch generative adversarial networks with edge detection image inpainting (MPGE) was proposed. #3: That’s it, Done…! it’s protable.Image inpainting with large missing blocks is quite challenging to obtain visual consistency and realistic effect.
INPAINT 6.0 KEY HOW TO
How to Crack, Register or Free Activate Inpaint
INPAINT 6.0 KEY SERIAL KEY

In fact, such as picture cutout, watermark remove, and minor defect elimination or improvement, Teorex’s Inpaint is enough.
INPAINT 6.0 KEY SOFTWARE
But if you are going to learn the complex and large graphics processing software (such as Photoshop) only in order to achieve these small changes, i think there is no need to make a big deal out of it. Inpaint Serial key If you need to modify pictures as mentioned above, many image processing software can meet your needs. Inpaint Crack Many wallpapers or images downloaded from the Internet may have watermarks Or your photographs, pictures have some defects Or some contents need to be covered or removed.
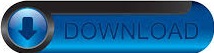